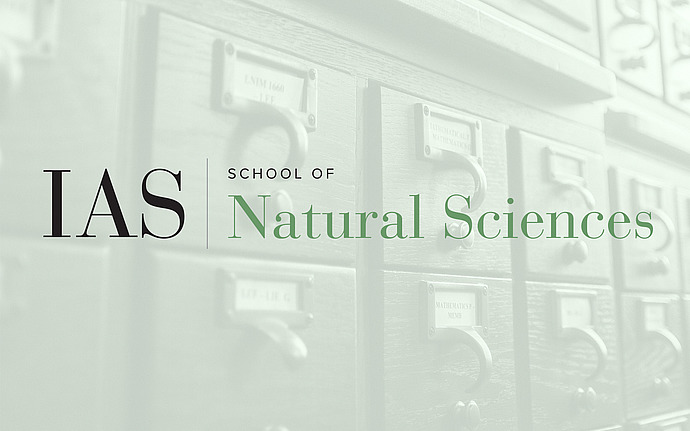
Princeton University Data Science / COMPASS Seminar
Stream Detection Using Photometric Distance Posteriors Deep-Learned from Gaia
I will present a novel technique to automatically associate stars to stellar streams using photometry and 4D kinematic information from Gaia DR2. We use HDBSCAN for clustering stars into stream candidates using a custom distance metric learned with neighborhood component analysis (NCA) on mock stream data from FIRE simulations, in combination with a distance filter on photometric distances from a data-driven model. Without tuning or prior information about the existence or kinematics of GD-1, the algorithm is able to detect GD-1 in raw Gaia data automatically. In parallel, I will present the methodology used to create a catalog of photometric distance posteriors derived from a data-driven model using Gaia parallaxes as a training set. Dust estimation and dereddening is done iteratively inside the model. Full posterior densities are given rather than point estimates, allowing for MCMC integration over stellar type and dust ambiguities. Finally, I will discuss additional applications for this catalog.Every week we discuss data science methods and applications from papers, reviews, software releases, etc. We also have demos for useful/fancy methods by locals and visitors through the COMPASS program. The setting is informal. Material should be presented directly from the source or on the white board, demos should be hands-on. We collect links to documents, source code, tutorials, etc. for later perusal in this github repo. If you would like to present at the data science seminar, please contact the organizers Peter Melchior (melchior[at]astro.princeton.edu), Adrian Price-Whelan (adrn[at]astro.princeton.edu), Christina Kreisch (ckreisch[at]astro.princeton.edu), Lachlan Lancaster (lachlanl[at]princeton.edu).
Date & Time
February 18, 2019 | 4:00pm – 5:00pm
Location
Peter B. Lewis Science Library, Visualization Lab, Rm. 347Speakers
Miles Cranmer
Affiliation
Princeton University