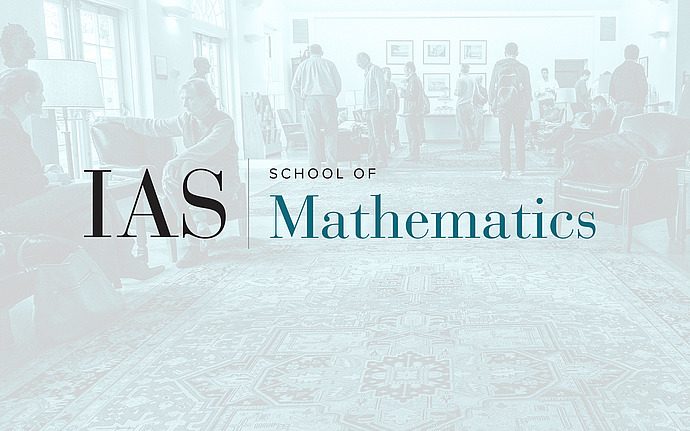
Theoretical Machine Learning Seminar - PCTS Seminar Series: Deep Learning for Physics
Understanding Machine Learning via Exactly Solvable Statistical Physics Models
Please Note: The seminars are not open to the general public, but only to active researchers.
Register here for this event: https://docs.google.com/forms/d/e/1FAIpQLScJ-BUVgJod6NGrreI26pedg8wGEyPhh3WMDskE1hIac_Yp3Q/viewform
The affinity between statistical physics and machine learning has long history, this is reflected even in the machine learning terminology that is in part adopted from physics. I will describe the main lines of this long-lasting friendship in the context of current theoretical challenges and open questions about deep learning. Theoretical physics often proceeds in terms of solvable synthetic models, I will describe the related line of work on solvable models of simple feed-forward neural networks. I will highlight a path forward to capture the subtle interplay between the structure of the data, the architecture of the network, and the learning algorithm.
Date & Time
Location
Jadwin Hall PCTS Seminar Room 407 (Princeton University)Speakers
Affiliation
Categories
Notes
Each talk will be preceded with lunch at 11:45 am. The talks will be held from 12:25-1:30 pm and from 2:00 - 3:00 pm.