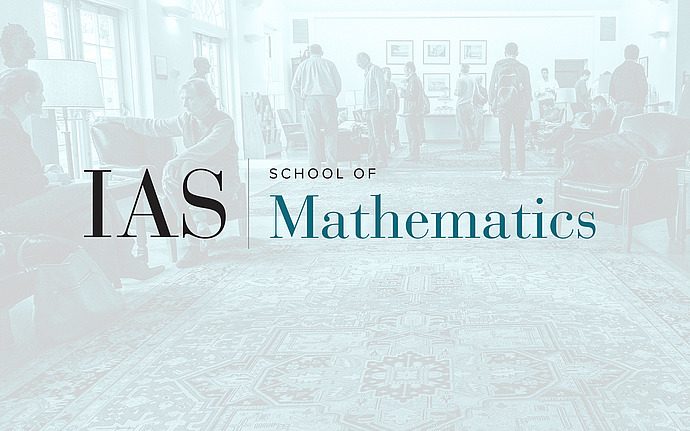
Theoretical Machine Learning Seminar
Meta-Learning: Why It’s Hard and What We Can Do
Meta-learning (or learning to learn) studies how to use machine learning to design machine learning methods themselves. We consider an optimization-based formulation of meta-learning that learns to design an optimization algorithm automatically, which we call Learning to Optimize. Surprisingly, it turns out that the most straightforward approach of learning such an algorithm, namely backpropagation, does not work. We explore the underlying reason for this failure, devise a solution based on reinforcement learning and discuss the open challenges in meta-learning.
Date & Time
April 09, 2020 | 3:00pm – 4:30pm
Location
https://theias.zoom.us/j/384099138Speakers
Affiliation
Member, School of Mathematics