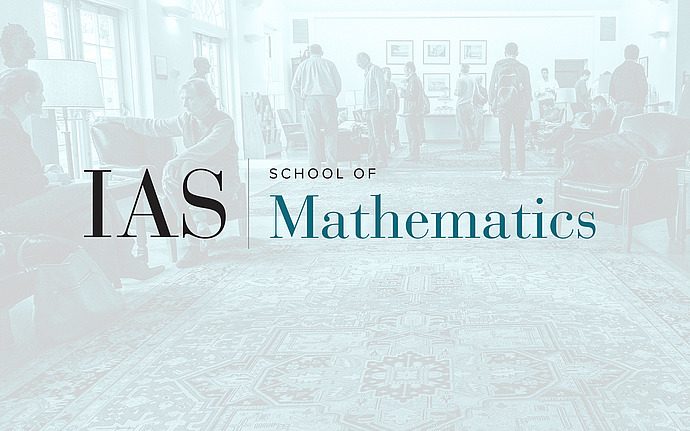
Theoretical Machine Learning Seminar
A Fourier Analysis Perspective of Training Dynamics of Deep Neural Networks
This talk focuses on a general phenomenon of "Frequency-Principle" that DNNs often fit target functions from low to high frequencies during the training. I will present empirical evidences on real datasets and deep networks of different settings as well as theoretical results about Frequency-Principle. Specifically, I will talk about a "Linear Frequency-Principle" dynamics derived from two-layer neural networks at the NTK regime, which quantifies the priority of different frequencies during the training and gives rise to an apriori generalization error bound based on the frequency composition of the target function.
Date & Time
November 26, 2019 | 11:30am – 12:30pm
Location
White-LevySpeakers
Affiliation
Member, School of Mathematics