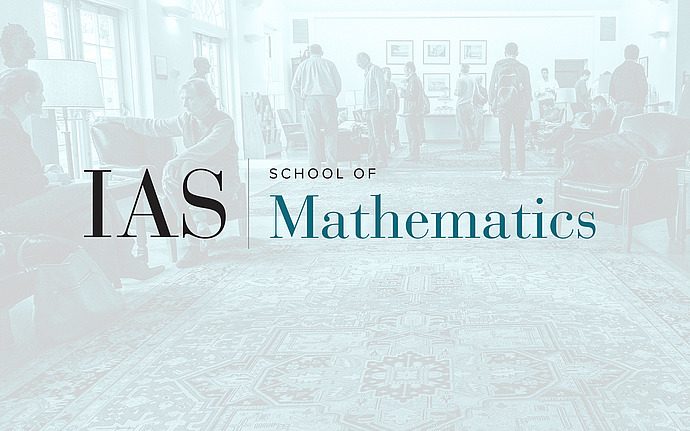
Theoretical Machine Learning Seminar
Generalized Framework for Nonlinear Acceleration
Nonlinear Acceleration Algorithms, such as BFGS, were widely used in optimization due to their impressive performance even for large scale problems. However, these methods present a non negligeable number of drawbacks, such as a strong lack of theoretical guarantees and the requirement of a line-search to ensure convergence. Recently, Scieur et al. proposed a regularized version of Anderson Acceleration which achieves an asymtoptically optimal rate of convergence on smooth and strongly convex functions, but it is now unclear how to extend this idea to other nonlinear acceleration algorithms.In this work, we propose a unified analysis for nonlinear acceleration methods in the quadratic case, which links Broyden Type-I and Type-II methods, BFGS and DFP formulas, Anderson Acceleration and Conjugate Gradients method. This is a step toward a generalized class of regularized nonlinear acceleration algorithms with provable convergence guarantees.