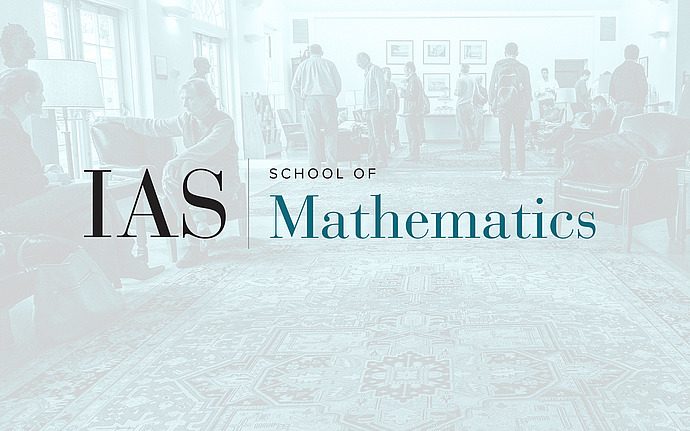
Theoretical Machine Learning Seminar
Online Improper Learning with an Approximation Oracle
We revisit the question of reducing online learning to approximate optimization of the offline problem. In this setting, we give two algorithms with near-optimal performance in the full information setting: they guarantee optimal regret and require only poly-logarithmically many calls to the approxi- mation oracle per iteration. Furthermore, these algorithms apply to the more general improper learning problems. In the bandit setting, our algorithm also significantly improves the best previously known oracle complexity while maintaining the same regret.
Joint work with Elad Hazan, Wei Hu, Yuanzhi Li.
Date & Time
April 19, 2018 | 12:15pm – 1:45pm
Location
White-Levy RoomSpeakers
Zhiyuan Li
Affiliation
Princeton University