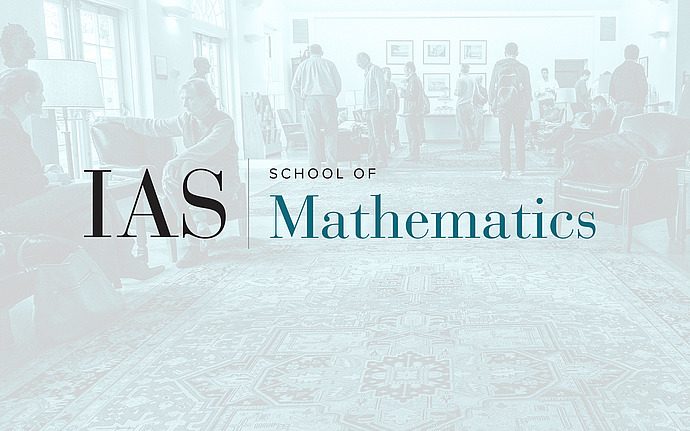
Theoretical Machine Learning Seminar
Stability and Generalization in Adaptive Data Analysis
Datasets are often used multiple times with each successive analysis depending on the outcomes of previous analyses on the same dataset. Standard techniques for ensuring generalization and statistical validity do not account for this adaptive dependence. In this talk I will overview a recent line of work on adaptive data analysis that studies the problem of answering a sequence of "queries" about the data distribution where each query may depend arbitrarily on answers to previous queries. I'll then focus on a new notion of algorithmic stability with the important property that it "composes" well when data is reused. I'll demonstrate that this notion of stability implies that the algorithm reveals little information about its input and, in particular, cannot lead to overfitting. Finally, I'll describe simple algorithms based on this notion that can answer statistical queries about the dataset with substantially improved accuracy guarantees for low-variance queries.
Based in part on a joint work with Thomas Steinke.