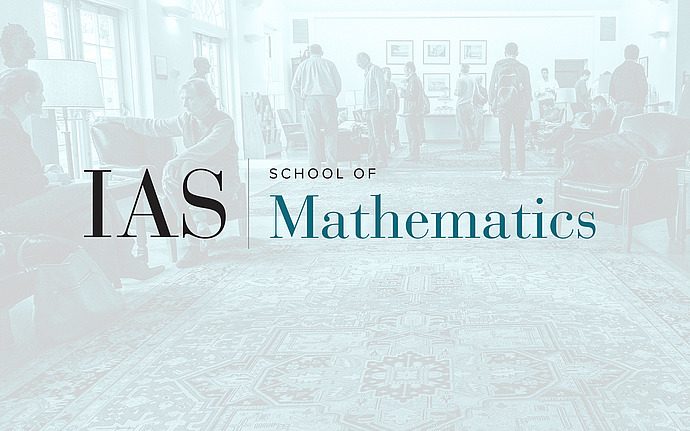
Computer Science/Discrete Mathematics Seminar I
Some provable bounds for deep learning
Deep learning, a modern version of neural nets, is increasingly seen as a promising way to implement AI tasks such as speech recognition and image recognition. Most current algorithms are heuristic and have no provable guarantees. This talk will describe provable learning of an interesting class of deep networks which are neural nets. Here a deep net is viewed as a generative model for a probability distribution on inputs, using the "denoising autoencoder" framework of Vincent et al. The talk will be self-contained. (Joint work with Aditya Bhaskara, Rong Ge, Tengyu Ma)
Date & Time
September 30, 2013 | 11:15am – 12:15pm