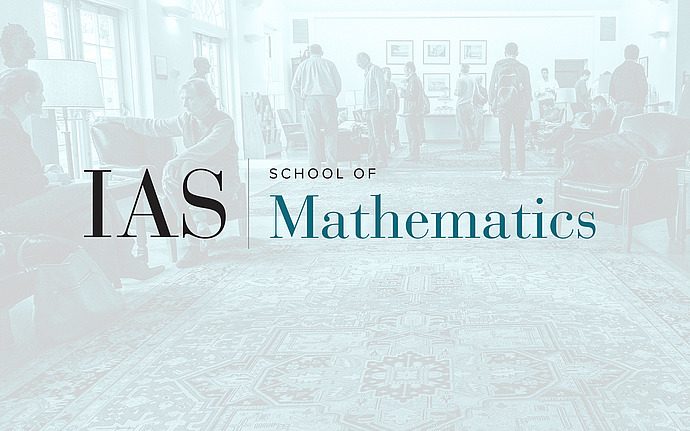
Computer Science/Discrete Mathematics Seminar I
Learning and Testing k-Model Distributions
A k-modal probability distribution over the domain {1,...,N} is one whose histogram has at most k "peaks" and "valleys". Such distributions are a natural generalization of the well-studied class of monotone increasing (or monotone decreasing) probability distributions. We study the problem of learning an unknown k-modal distribution from samples. We also study a related problem in property testing: given access to samples from an unknown k-modal distribution p, determine whether p is identical to q or p is "far" from q. Here q is a k-modal distribution which may be given explicitly or may be available via sample access. We give algorithms for these problems that are provably close to the best possible in terms of sample and time complexity. An interesting feature of our approach is that our learning algorithms use ingredients from property testing and vice versa. Joint work with Costis Daskalakis and Ilias Diakonikolas.