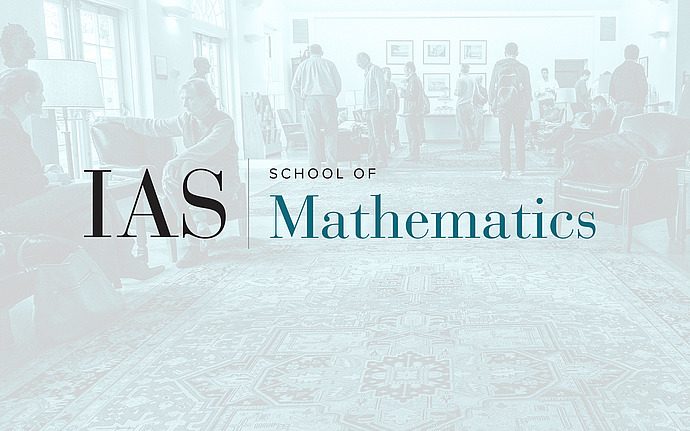
Theoretical Machine Learning Seminar
Learning-Based Sketching Algorithms
Classical algorithms typically provide "one size fits all" performance, and do not leverage properties or patterns in their inputs. A recent line of work aims to address this issue by developing algorithms that use machine learning predictions to improve their performance. In this talk I will present two examples of this type, in the context of streaming and sketching algorithms. In particular, I will show how to use machine learning predictions to improve the performance of (a) low-memory streaming algorithms for frequency estimation, and (b) generating space partitions for nearest neighbor search.
The talk will cover material from papers co-authored with Y Dong, CY Hsu, D Katabi, I Razenshteyn, T Wagner and A Vakilian.
Date & Time
Location
Remote Access Only - see link belowSpeakers
Affiliation
Event Series
Categories
Notes
We welcome broad participation in our seminar series. To receive login details, interested participants will need to fill out a registration form accessible from the link below. Upcoming seminars in this series can be found here.