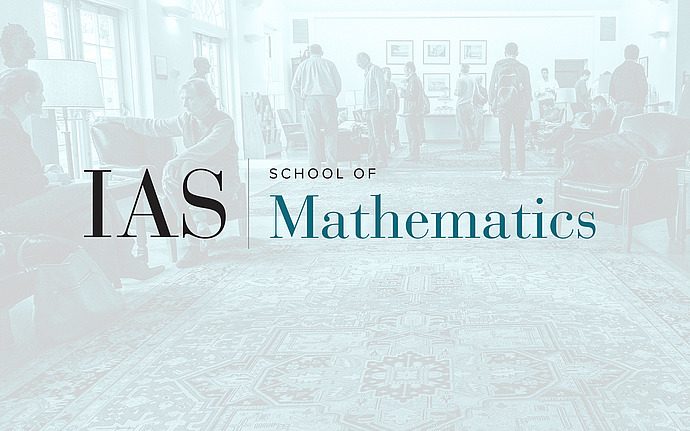
Members’ Seminar
Direct and dual Information Bottleneck frameworks for Deep Learning
The Information Bottleneck (IB) is an information theoretic framework for optimal representation learning. It stems from the problem of finding minimal sufficient statistics in supervised learning, but has insightful implications for Deep Learning. In particular, its the only theory that gives concrete predictions on the different representations in each layer and their potential computational benefit. I will review the theory and its new version, the dual Information Bottleneck, related to the variational Information Bottleneck which is gaining practical popularity. In particular, I will discuss the implications of the critical points (phase transitions) of the IB and dual IB and their importance for topological transformations of the consecutive successively refinable representations. Based on joint works with Ravid Schwartz Ziv, Noga Zaslavsky, and Zoe Piran.